Mid-2016 Tor bug retrospective, with lessons for future coding
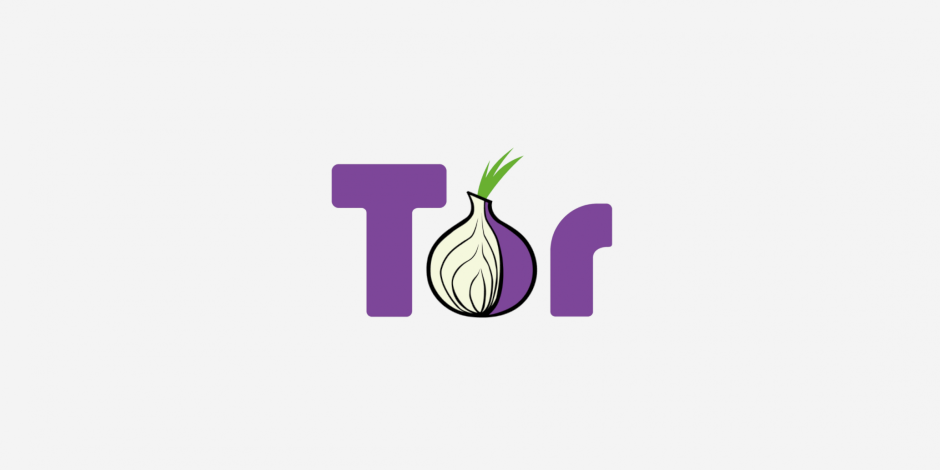
I. Introduction
Programs have bugs because developers make mistakes. Generally, when we discover a serious bug, we try to fix it as soon as we can and move on. But many groups have found it helpful to pause periodically and look for trends in the bugs they have discovered or fixed over the course of their projects. By finding trends, we can try to identify ways to develop our software better.
I recently did an informal review of our major bugs from the last few years. (I'm calling it "informal" rather than "formal" mainly because I made up the process as I went along.)
My goals were to see if we're right in our understanding of what causes bugs in Tor, and what approaches to avoid bugs and limit their impact would be most effective.
By reviewing all the bugs and looking for patterns, I'm hoping that we can test some of our operating hypotheses about what allows severe bugs to happen, and what practices would prevent them. If this information is reasonably accurate, it should help us use our time and resources more effectively to write our code more safely over the coming years.
II. Methodology
I took an inventory of "severe bugs" from three sources:
- Tickets in the Tor bugtracker with high priority, closed in 0.2.5.x or later.
- Tickets in the Tor bugtracker with high severity, closed in 0.2.5.x or later.
- An assessment of bugs listed as changelogs for 0.2.5.x and later.
For each of these cases, I assessed "is this severe" and "is this really a bug" more or less ad hoc, erring on the side of inclusion. I wound up with 70 tickets.
At this point, I did a hand-examination of each ticket, asking these questions:
- What was the bug?
- What was the impact?
- Why did the bug happen? What might have prevented it or lowered its impact? What might have detected it earlier?
I then used a set of keywords to group tickets by similar causes or potential prevention methods.
Finally, I grouped tickets by keywords, looking for the keywords that had the largest number of tickets associated.
Limitations of this methodology.
Consider this an exploratory exercise, not a scientific finding. We should look into formalizing the methodology more and giving it more process the next time we do it, for these reasons:
- It's entirely dependent on my judgment of "is this severe" and "is this a bug."
- The categories were made up as I went along.
- Many of the hypotheses it tests are post-hoc hypotheses.
- I haven't done very much checking on the input data yet; I wouldn't consider this scientific till somebody else has looked for bugs I missed and analyses I got wrong. There's no reason to think that I got these particularly right.
- The only objective measure I'm using is "how many bugs did I tag with a given keyword?," with the assumption that any keyword covering a lot of bugs is particularly important. But that's based on a semi-subjective assessment (tags), applied to a semi-subjective population ("bugs" I judged "severe"), and ignores bug impact.
III. Results and recommendations
1. Testing is helpful (big surprise).
We've believed for a while that we can reduce the number of bugs that make it into the wild by using more tests on our codebase. This seems broadly true, but incomplete.
First, it seems that only about half of our severe bugs appeared to be the kind of thing that better tests would have caught. The other half involved logic errors and design oversights that would probably have made it through testing.
Second, it seems that in some cases, our existing tests were adequate to the job, if only we had automated them better, or had run them more consistently, more rigorously, or under more conditions.
In all cases, of course, automation isn't quite enough. We must also have the automated tests run regularly (daily?), and make sure that the results are available to developers in a convenient way.
Recommendation 1.1 Run our automated unit tests under more code-hardening methodologies.
This includes --enable-expensive-hardening under GCC and clang, valgrind with leak checking turned on, and anything else we can find.
Bugs where running tests under hardening or valgrind might have helped include: #13104, #14821, #17401, #17404, #18454.
Recommendation 1.2: Also run test-network and test-stem in an automated environment.
These checks can detect a lot of problems, but right now we only try the stem tests in automated builds, and we don't try them with hardening.
Cases where a suitably extended (or completely vanilla) stem or chutney test case might have helped include: #8746, #9296, #10465, #10849, #11200, #13698,
#15245, #15801, #16247, #16248, #17668, #17702, #17772, #18116, #18318, and
#18517.
Recommendation 1.3: Automate use of static analysis tools with Tor.
There were some cases where we found a bug using a static analysis tool later than we might have, because the static analysis tool had to be hand-launched. We can get faster bug resolution by automatically running all the static analysis tools we use. (We've already done this.)
Static analyzers might have caught: #13477 and #18454, at very little effort on our part.
Recommendation 1.4: Continue requiring unit tests for new code, and writing unit tests for old code.
Untested code had bugs at a higher rate than tested code.
Bugs where plain old unit tests might have helped include: #11824,
#12195, #13066, #13151, #15083, #16400, #17041, #17668, #17702, #17772,
#17876, #18162, and #18318.
Recommendation 1.5: Get more users to try out our nightly builds.
Having more users of our nightly builds would help us notice more bugs on the git master branch before those bugs appear in stable or alpha releases.
Having users for our nightly builds would have prevented #11200 entirely.
Recommendation 1.6: Whenever possible, write integration tests for new features.
Features that lack integration tests via Chutney or some other mechanism tend to have bugs that last longer than other bugs before anybody notices them.
(See stem/chutney list above.)
Recommendation 1.7: We need more tests about shutting down busy clients and relays.
Our code tends to have a fair number of corner cases concerning shutting down at the wrong time, and crashing or asserting rather than exiting cleanly.
Careful shutdown tests might have caught #8746 and #18116.
2. Which C difficulties are a problem in practice?
C is notoriously tricky, and we've put a fair amount of effort into avoiding its nastier failure modes. The C-related errors that did cause problems for us were not the ones I would have expected: Buffer overflows generally got caught very quickly. There are other C warts we've been less successful at avoiding.
Recommendation 2.1: Pay particular attention to integer overflow.
We had a few cases where signed integer overflow (or unsigned overflow) could cause bad bugs in our code, some resulting in heap corruption.
Perhaps we should prefer using unsigned integers everywhere we don't actually need signed integers? But although unsigned overflow isn't undefined behavior, it's still usually a bug when it's not intentional. So maybe preferring unsigned values wouldn't be so great.
Perhaps smartlists and similar data structures should use size_t internally instead of int for size and capacity. (Their use of int in their APIs isn't easy to change because of the rest of the codebase.)
Our work on using -ftrapv throughout our code by default (in #17983) should help turn subtle signed overflow errors into crashes.
Integer overflow was behind bugs #13104 and #18162.
Recommendation 2.2: Avoid void-pointer punning in API design; add more type-specialized APIs.
We have two styles of container: Those that are specialized for a given type, and those that store void*. In nearly all cases, the void* ones have involved programmers making mistakes about what type they actually contained, in some case causing hard-to-debug issues.
Bug #18454 is one example of a void-punning problem.
Recommendation 2.3: Continue to forbid new hand-written parsing code in C.
This caused fewer issues than you'd think (only a few ones for binary encoding and parsing, and only one for text parsing), but they were particularly troublesome.
Outside of hand-written parsing code, memory violations are less frequent than you'd think.
Examples of these bugs include #4168, #17668, #13151, #15202, #15601, #15823, and #17404.
Recommendation 2.4: In the long-term, using a higher-level language than C would be wise.
This is a longer term project, however, and would have to happen after we get more module separation.
Bugs that would be more difficult (or impossible) to cause in a safe language include: #9296, #9602, #11743, #12694, #13104, #13477, #15202, #15823,
#15901, #17041, #17401, #17404, #18162, and #18454.
3. State machines, object lifetimes, and uncommon paths.
Many of our more subtle errors were caused by objects being in states that we didn't think they could actually be in at the same time, usually on error cases, shutdown paths, or other parts of the codebase not directly tied to the dominant path.
Recommendation 3.1: For every object type, we should have a very clear understanding of its lifetime, who creates it, who destroys it, and how long it is guaranteed to last.
We should document this for every type, and try to make sure that the documentation is simple and easy to verify.
We could also consider more reference-counting or handles (qv) to avoid lifetime problems.
Bugs related to object lifetime include: #7912, #8387, #8746, #9602, #11743,
#17041, #17401, #17752, #18116, and #18251.
Recommendation 3.2: State machines, particularly in error handling, need to be documented and/or simplified.
We have numerous implicit state machines scattered throughout our codebase, but few of them are explicitly expressed or documented as state machines. We should have a standard way to express and create new state machines to ensure their correctness, and to better analyze them.
Bugs related to unclear state machines include: #7912, #8387, #8746, #9645,
#13698, #15245, #15515, #16013, #16260, and #17674.
Recommendation 3.3: Design with error handling in mind.
This might be as simple as documenting state machines and noticing cases where transitions aren't considered, or might be more complicated.
Error handling bugs include: #8746, #9645, #9819, #10777, #13698, #16360,
#17041, and #17674.
4. Assertion problems (too many, too few).
Recommendation 4.1: Assert less; BUG more.
A great many of our crash bugs were caused by assertions that did not actually need to be assertions. Some part of the code was violating an invariant, but rather than exiting the program, we could have simply had the function that noticed the problem exit with an error, fix up the invariant, or recover in some other way.
We've recently added a family of nonfatal assertion (#18613) functions; we should use them wherever reasonable.
Bugs related to overzealous assertions include #9602, #10465, #15083, #15601,
#15776, #16013, #16248, #16400, and #18116.
5. Keeping backward compatibility with broken things for too long.
Recommendation 5.1: all backward compatibility code should have a timeout date.
On several occasions we added backward compatibility code to keep an old version of Tor working, but left it enabled for longer than we needed to. This code has tended not to get the same regular attention it deserves, and has also tended to hold surprising deviations from the specification. We should audit the code that's there today and see what we can remove, and we should never add new code of this kind without adding a ticket and a comment planning to remove it.
Less focus on backward compatibility would have prevented bugs #1038, bug
#9777, and bug #13426.
Recommendation 5.2: Examine all XXX, TODO, and FIXME code.
In several cases, the original author of a piece of buggy code scheduled it for removal with an "XXX02..." comment, or noted some potential problem with it. If we had been more vigilant about searching for and categorizing these cases, we could have removed or fixed this obsolete code before it had caused severe bugs.
There are 407 instances of XXX, TODO, ???, FFF, or FIXME in src/common and src/or right now; we should get on auditing those and recategorizing them as "fix now, open a ticket", "fix later, open a ticket", or something else.
Bugs of this kind include #1038, #11648, and #17702.
6. Several errors are related to unrelated spec mismatches.
I don't have a firm set of conclusions here, other than to maybe make sure that our tests specifically correspond to what the spec says?
7. Too many options.
Recommendation 7.1: Cull the options; remove ones we don't need/use/advise.
Several severe bugs could only occur when the user specifically set one or more options which, on reflection, nobody should really set. In most cases, these options were added for fairly good reasons (such as protocol migration or bug workarounds), but they no longer serve a good purpose.
We should go through all of our settings and see what we can disable or deprecate. This may also allow us to get rid of more code.
Bugs caused by seldom-used or ill-advised settings for options include:
#8387, #10849, #15245, #16069, and #17674.
Recommendation 7.2:Possibly, deprecate using a single Tor for many roles at a time.
Many bugs were related to running a HS+relay or HS+client or client+relay configuration. Maybe we should recommend separate processes for these deployment scenarios.
#9819 is one bug of this kind.
8. Release cycle issues.
Recommendation 8.1: Tighten end-of-cycle freezes issues.
Large features merged at the end of release, predictably, caused big bugs down the line.
The discussion and history on bugs #7912 and #10777 indicate that waiting until very late in the cycle was probably not as good an idea as it seemed at the time.
9. Conventions.
Recommendation 9.1: We should favor a single convention for return values, and not accept code that doesn't follow it.
We have had a few bugs caused by differing return-value conventions on similar functions. Our most common convention has been to have a negative value indicate failure and zero to indicate success. When 0 indicates failure and positive values indicate success, we usually can expect to have a bug in the calling code someplace.
Bug #16360 was caused by a misunderstanding of this kind, and could have been much worse than it really was.
10. Complexity.
Recommendation 10.1: Callgraph complexity hits us a lot -- particular in code where a calling function assumes that a called function will not make certain changes in other structures.
We should, whenever possible, simplify our callgraph to remove cycles, and to limit maximum call depth.
Bugs resulting from, or worsened by, complexit y in the callgraph, include
#4900, #13698, #16013, and #17752.
Recommendation 10.2: Prefer identify-loop-then-modify-loop to all-operations-at-once-loop.
Several hard-to-diagnose bugs were called by code where we identified targets for some operation and simultaneously performed that operation. In general, we should probably have our default approach involve identifying the items to operate on first, and operating on them afterwards. We might want to operate on them immediately afterwards, or schedule the operation for higher in the mainloop.
Bugs #16013 and #17752 were caused by the modify-in-iterator pattern.
Recommendation 10.3: Perhaps we should have a container-freezer.
We have code that supports removing a member from a smartlist or hashtable while iterating over it.... but adding or removing members through other means at the same time won't work. What's more, debugging the results is annoyingly difficult. Perhaps we should have our code catch such attempts and give an assertion failure.
Bugs #16013 and #17752 were caused by the modify-in-iterator pattern.
Recommendation 10.4: Duplicated code is trouble; different functions that do the same thing differently are trouble.
This is well-known, but nonetheless, we have a few cases where we grew two functions to do similar things, patched one to solve a problem or add a feature, but forgot to patch the other.
Code duplication was at issue in bugs #12195, #13066, and #17772.
11. Target areas.
Recommendation 11.1: Our path-selection/node-selection code is very complex, and needs more testing and rewrites.
More than in most other areas, we found bugs in the code that selects paths and nodes. This code is hard to test in part because it's randomized, and in part because it looks at several global structures including the nodelist, the microdescriptor set, the networkstatus, and state related to guard nodes. We should look at ways to simplify our logic here as much as possible.
This code was related to bugs #9777, #10777, #13066, #16247, #17674, and
#17772.
IV. Next steps
Some time over the next month or so, we should re-scan this document for actionable items to improve our future code practices, create bugtracker tickets for those items, and try to sort them by their benefit-to-effort ratio. We should also make a plan to try this again in a year or two.
Comments
Please note that the comment area below has been archived.
"unit tests tests" in
"unit tests tests" in reco.1.4
Thanks; fixed!
Thanks; fixed!
reco.2.4: get ready for
reco.2.4: get ready for C/C++ unification in 2017.
Thank you nickm and all Tor
Thank you nickm and all Tor developers for your tireless efforts to improve Tor. Your focus, dedication, and genius are well established, so it is truly amazing how you continue to hone your work and challenge assumptions. I am really looking forward to seeing how these strategic efforts lead to even more advances. The world is changing so fast, and it is comforting to know that Tor is consolidating its gains and planning for even more success!
F*cking Ukrainian exit nodes
F*cking Ukrainian exit nodes return blank pages instead of Russian sites (.ru)!!!
Does this have something to
Does this have something to do with the bug retrospective I posted?
No. It's just yet another
No. It's just yet another person complaining about something related to the Tor network as a whole on a generic blog post. He might as well have just said "ToR is backdoored by the NSA and insecure. I heard it on the news", or something of the sort, and it would be equally on-topic.
"complexit y" in reco.10.1
"complexit y" in reco.10.1
Recommendation 10.4: "Code
Recommendation 10.4: "Code duplication was at issue in bugs" ? )
https://github.com/TrustInSof
https://github.com/TrustInSoft/tis-interpreter
Or just stick with something
Or just stick with something truly open-source, you know.. :)
http://frama-c.com/
Do you plan to use formal
Do you plan to use formal verification to help you ensure critical parts of your code are in check ? Like annotating with E-ACSL (for Frama-C) or even turning to a dedicated language, like F* ?
Not currently, but it would
Not currently, but it would be interesting to see somebody give it a try. Right now, my sense is that formal verification in cryptographic software is getting most of its uptake at the lowest level, and less so in higher-level apps.
Some part of the code was
Some part of the code was violating an invariant, but rather than exiting the program, we could have simply had the function that noticed the problem exit with an error, fix up the invariant, or recover in some other way.
Careful there.
I do not like the sound of this. When you notice invariant violations you can be sure that your program is no longer what you'd think of as your program. It is the virtual machine for someone else's program.
I think the only sensible thing to do in such cases is to die in a pool of black blood. This is what I would consider failing safe. Given the alternative better inconvenience than insecurity.
However, I do recognise that in the case of Tor the problem is compounded. In less-sensitive environments one wouldn't worry too much about denial-of-service attacks. Yet, for Tor, DoS vulnerabilities can enable powerful correlation attacks (AFAICS).
Like being stuck between a rock and a hard place. But surely this is not a problem unique to Tor. It is common to all software of which high reliability and dependability is required, like safety-critical systems. So maybe that's a place to look for ideas?
I understand what you're
I understand what you're saying, but please have a look over some of the bugs mentioned in that section. For some of them, you'd actually want to crash, sure. But for others, a better response would have been to reject the object currently being parsed; or to close the connection being used; or to kill off the circuit in question.
Trends like this are a big part of the reason why I did this retrospective: to compare practice with theory. In theory, every assertion failure reflects a programming error from which no recovery is possible. And in a world where that's always true, there's no need to look more closely. But in practice, it sure does seem like a lot of the triggerable assertions we've encountered never actually needed to be assertions at all.
Your response is giving me
Your response is giving me the impression that what these assertions were checking weren't real invariants, and shouldn't have been treated as such.
Anyway, I'll have a look at those tickets. Cheers!
Rust anyone? Memory
Rust anyone? Memory protections, reduced code complexity, and near C level speed should make the transition a no-brainer. There's a promising project from the Maidsafe foundation that courageously (considering crowdsale/investor pressure) delayed the their MVP to switch the entire codebase from C++ to Rust in about half a year.
Roughly 500k lines down to about 30k with extended functionality and greater maturity. I can't think of anyone better than Nick to get the wagon going. The man's a wizard. Tossing up one of Tors' modules in Rust should be a breeze for that guy.
IMO, anything that retains the intended functionality of Tor while reducing the complexity, preventing memory leaks, and shrinking the code base deserves further investigation. This would make it easier for the community to contribute and expedite development of countermeasures against the ever tightening noose of blanket surveillance and censorship.
I implore some wise soul with the experience and skill set to devote a weekend to porting at least one module to Rust. Get your feet wet for all of our sake.
I like rust too, but the
I like rust too, but the rewrite project is going to be tricky from what I can tell of rust. (Not impossible -- just a lot of work to get started on.)
One of our big problems right now is that our modules are not well-isolated. Nearly every C file in src/or is reachable from nearly every C file. So in order to rewrite a single module in rust, we'd have to not only provide a C-to-rust bridge for using that module, but also a correctly lifetime-annotated rust-to-C bridge for every other module which that module can use.
Also, from what I've been able to tell, integration between rust and automake is not really there yet, in a way where we could write our project in a mix of the two.
Both of those problems are solveable. I did a lot of work last year to improve our callgraph: there used to be a huge blob of 450 functions every one of which could reach all the others. Now that number is down under 20. I think that with a similar amount of tooling and refactoring, we can turn the inter-module callgraph into something manageable, and make the idea of modules as isolated pieces more sustainable.
And the build stuff should just be a simple matter of scripting and writing HOWTO documents, for "some wise soul with the right experience and skill set". Though I'd expect to have a few rounds of communication and misunderstanding before we figured out how we wanted an integrated build process to actually work.
(disclaimer: I have written about 100 lines in rust, but only about 100 lines.)
The blogpost at
The blogpost at https://thesquareplanet.com/blog/the-path-to-rust/ discusses what happens if Tor were written in Rust. It seems Rust catches at least some of the bugs.
@ Nick: I don't know how you
@ Nick:
I don't know how you found the time to do this, but I'm very glad you did!
> I recently did an informal review of our major bugs from the last few years. (I'm calling it "informal" rather than "formal" mainly because I made up the process as I went along.)
I think that's wise: given the urgency and lack of benchmarks, just plunge in and start trying to get some initial sense of what TP might possibly do to try to decrease the likelihood of bugs, especially those which might have a devastating effect.
> We should look into formalizing the methodology more and giving it more process the next time we do it.
Yes.
How sophisticated is your automated code auditing?
> In several cases, the original author of a piece of buggy code scheduled it for removal with an "XXX02..." comment, or noted some potential problem with it. If we had been more vigilant about searching for and categorizing these cases, we could have removed or fixed this obsolete code before it had caused severe bugs.
Could "AI" methods which operate on "unstructured text" help track this kind of thing?
(Our enemies are using AI methods to attack us; we should consider using it, cautiously and only after due consideration of the failure modes of Bayesian inference, to help defend ourselves.)
> When 0 indicates failure and positive values indicate success, we usually can expect to have a bug in the calling code someplace.
Oh, snap, that's also one of the most common sources of errors for statisticians trying to perform Bayesian statistical analysis on government data! Forgetting to recode data obtained from some Big Data repository before performing conditional probability queries to deanonymize bad guys involved in drawing up target lists for USG drone strikes, etc.
> We should, whenever possible, simplify our callgraph to remove cycles,
In Bayesian networks, USIC operatives confront a dual problem: the formalism prohibits cycles, but they need them to model feedback loops (if USG kills X, family and friends and government of X react, USG reacts to their reactions with further killings, etc).
Have you thought about graph theoretic quantities which might be useful to quantify the kind of complexity you want to reduce?
> This code is hard to test in part because it's randomized,
This is also a huge problem for people who worry about the legal and technical problems which are resulting from governments all over the world augmenting (or replacing) human bureaucrats with AI-type algorithms exploiting Big Data sources to make decisions about continual governmental "interventions" in the life of each citizen (from various choices, which one maximizes benefit to the government over the next decade?). As you know, many of the most efficient and thus most-used algorithms for a huge range of tasks are nondeterministic, which means that it can be very hard to understand how they interact. You can try running more simulations (using more AI tools!) but each run might give wildly divergent results. Far from curing cancer or crime, the result is even more confusion. And thus, even more misleading scientifically useless academic papers, or even worse, devastating state-sponsored disruption of the lives of millions of ordinary citizens.
Sounds like you are dealing with issues where probability meets relations meets first order logic (e.g. there is a logical statement about your program's variables which you want to ensure always remains true). You probably know that there is currently considerable excitement in AI about the new field of Markov logic, which attempts to provide a theoretical framework for handling such issues, maybe even to help solve real world problems like making Tor better.
USG is using statistical relational learning to help the bad guys to attack everyone in the world; maybe the same ideas can be used to help defend us.
> Our path-selection/node-selection code is very complex, and needs more testing and rewrites.
Yes, this should be a priority. No idea how you'll find the time, but I hope you can!
I'm not sure what you mean
I'm not sure what you mean by AI. The methods of automated analysis which people are using are called static analysis and dynamic analysis, or fuzzing. Neither of those are considered artificial intelligence, even though some of the most advanced dynamic analysis tools such as AFL (American Fuzzy Lop) involve instrumented fuzzing which is based on intelligent feedback from the internal state of the program as it is being executed.
Static analysis involves feeding the source code (or in some cases, the bytecode) into a program which attempts to predict unintended behavior without actually executing the program. It uses heuristics to look for basic things like typos, as well as following the data flow until it finds bugs or poor programing practice. Dynamic analysis involves executing the target program, and feeding it with bogus input until it crashes. Normally, invalid input is simply discarded, but when a bug is present, the invalid input results in a crash or other interesting behavior. The best dynamic analysis tools will inspect the internal behavior of the program even when it does not crash, so it can customize the bogus input to exercise all the code paths it can.
Tor Project uses static analysis extensively. It uses a bunch of different tools, as well as gcc's internal static analysis capabilities. I don't think they use dynamic analysis much, but I'm sure some people have used it for Tor. It's more difficult to do dynamic analysis against networking programs, though still certainly possible
I do hope that Tor Project, in the future, develops a suite for utilizing AFL for fuzzing Tor. I imagine that would end up very beneficial.
Thank you for your
Thank you for your informative and clear reply!
> I'm not sure what you mean by AI.
I believe that we are talking about different things. I don't have any links handy, but I've been reading USG documents from various sources, including proposals from agencies like DARPA, which show strong USG interest in using "AI" techniques (I agree this buzzword is somewhat misleading given the current state of what might better be called "machine learning") to look for specific types of information in "unstructured text". Nick's statement
> In several cases, the original author of a piece of buggy code scheduled it for removal with an "XXX02..." comment, or noted some potential problem with it. If we had been more vigilant about searching for and categorizing these cases, we could have removed or fixed this obsolete code before it had caused severe bugs
seemed to me to resemble the kinds of problems I see DARPA type people wrestle with as they try to wean the USG off COBOL. However I lack hands on experience in attempting to address such issues, so I might be barking up the wrong tree.
Incidentally, I offer a plea to all reporters, bloggers, and policy makers, who are increasingly voicing concerns about the rapid expansion of AI, especially when government decisions about actions affecting individual citizens is increasingly made by some poorly understood (and possibly poorly coded) algorithm using unverified (and no doubt often "dirty") data drawn from numerous Big Data repositories: download some examples of neural net software, Bayesian predictive analysis software, and try working through some tutorials. Try them first on data sets where they give impressive results, then on some of your own data. IMO, this is the best way, maybe the only way, to begin to understand the limitations of these powerful tools.
Software has become so important for every aspect of modern life, including government, that a solid knowledge of probability theory, statistics, and experience using software algorithms for Bayesian predictive analysis, classification, etc., should really be required for a career in politics or journalism. *All* journalism should be "data journalism". And *all* politicians should be data scientists. Ideally.
I do of course realize that the reality is very different: aging Supreme Court justices and Senators and presidential candidates who trumpet their inability to use a laptop for even the simplest task and who boast of their ignorance of mathematics, as if that were somehow a good thing.
> people who worry about the
> people who worry about the legal and technical problems which are resulting from governments all over the world augmenting (or replacing) human bureaucrats with AI-type algorithms exploiting Big Data sources to make decisions about continual governmental "interventions" in the life of each citizen (from various choices, which one maximizes benefit to the government over the next decade?). As you know, many of the most efficient and thus most-used algorithms for a huge range of tasks are nondeterministic, which means that it can be very hard to understand how they interact. You can try running more simulations (using more AI tools!) but each run might give wildly divergent results. Far from curing cancer or crime, the result is even more confusion. And thus, even more misleading scientifically useless academic papers, or even worse, devastating state-sponsored disruption of the lives of millions of ordinary citizens.
Fabulous new article coauthored by Julia Angwin (author of Dragnet Nation):
http://www.motherjones.com/politics/2016/05/machine-police-future-crime…
The Legal System Uses an Algorithm to Predict If People Might Be Future Criminals. It Biased Against Blacks.
And it's terrible at predicting future crimes.
Julia Angwin, Jeff Larson, Surya Mattu and Lauren Kirchner, ProPublica
23 May 2016
> In 2014, then U.S. Attorney General Eric Holder warned that the risk scores might be injecting bias into the courts. He called for the U.S. Sentencing Commission to study their use. "Although these measures were crafted with the best of intentions, I am concerned that they inadvertently undermine our efforts to ensure individualized and equal justice," he said, adding, "they may exacerbate unwarranted and unjust disparities that are already far too common in our criminal justice system and in our society."
The Obama administration has published a variety of white papers, such as the "Podesta report" on Big Data, drawing attention to the danger that corporate abuse of "risk scoring" based on Big Data repositories could create a New Jim Crow in America. I share that concern, but I am very alarmed that the administration has steadfastly insisted that the authors of papers such as the Podesta report ignore the far greater dangers posed to the ordinary citizen by *government* misuse of "citizenship scores" and "threat scores" drawing on the far larger Big Data repositories available to agencies such as NSA, FBI, NCTC, often related to CVE (Countering Violent Extremism) "interventions" in the lives of American children as young as 3-7 years old. Holder's speech was a rare exception to this studied ignorance of the greater danger.
The reporters offer some striking anecdotal evidence that threat scores are leading the USG terribly astray:
> Yet something odd happened when Borden and Prater were booked into jail: A computer program spat out a score predicting the likelihood of each committing a future crime. Borden—who is black—was rated a high risk. Prater—who is white—was rated a low risk. Two years later, we know the computer algorithm got it exactly backward. Borden has not been charged with any new crimes. Prater is serving an eight-year prison term for subsequently breaking into a warehouse and stealing thousands of dollars' worth of electronics.
>
> [risk scores] are increasingly common in courtrooms across the nation. They are used to inform decisions about who can be set free at every stage of the criminal justice system, from assigning bond amounts—as is the case in Fort Lauderdale—to even more fundamental decisions about defendants' freedom. In Arizona, Colorado, Delaware, Kentucky, Louisiana, Oklahoma, Virginia, Washington and Wisconsin, the results of such assessments are given to judges during criminal sentencing.
But the reporters go much further and gathered and analyzed some statistics of their own:
> We obtained the risk scores assigned to more than 7,000 people arrested in Broward County, Florida, in 2013 and 2014 and checked to see how many were charged with new crimes over the next two years, the same benchmark used by the creators of the algorithm. The score proved remarkably unreliable in forecasting violent crime: Only 20 percent of the people predicted to commit violent crimes actually went on to do so. When a full range of crimes were taken into account—including misdemeanors such as driving with an expired license—the algorithm was somewhat more accurate than a coin flip. Of those deemed likely to re-offend, 61 percent were arrested for any subsequent crimes within two years.
Data journalism. To reporters everywhere I say: either you are using probability theory and statistics every working day, or you are simply not doing your job right.
More memory leak testing
More memory leak testing please ... right now Tor eats about 8-12GB of RAM per month in memory leaks on my setup (with the -alpha version -stable is worse). Besides being annoying, since the instance needs to be restarted at least monthly, this also causes user data to pile up (its an exit node, samples can be provided).
BC630CBBB518BE7E9F4E09712AB0269E9DC7D626
The fact that well-behaved
The fact that well-behaved web surfers who happen to use Tor are often treated as "bad actors" by automated "threat-detection" systems has been much discussed in this blog lately.
I have a question for Tor Project veterans (Roger, Nick) on a related point; Years ago on the tor-talk list, a poster who went by "Raccoon" mentioned the so-called Base Rate Fallacy for Bayes's Theorem: even if the ROC curve suggests that a risk scoring method is "highly accurate", if the events the method claims to predict are very rare in the general population, almost every event/person tagged with a high risk score will actually be incorrectly flagged.
The original discussion was in the context of cyberincident detection systems. Later Roger mentioned the fallacy in connection with a discussion in this blog of the probability theory relevant to a technical discussion of Tor protocols. (We're safe, we hope, from a certain kinds of automated deanonymization, because the fallacy kicks in.)
I'd like to raise this issue again in the context of calls in the past year by current Republican Party presidential candidate Donald Trump and former Democratic Party presidential candidate Wesley Clark for preventative detention of American Muslims who have been assigned high CVE "threat scores" by NCTC. FBI wants to assign such scores to every American schoolchild, and many US police departments assign "threat scores" to every citizen in their jurisdiction, so that cops know how itchy their trigger finger "should" be (according to the algorithm) when they respond to a call at your house.
Risk scoring software known as the COMPAS suite, which is sold by a company called Northpointe Inc, has been widely adopted in the US "justice system", where it is used to assess which inmates are liable to get into fights in prison, which prospective parolees should be deemed "too dangerous" to release early, etc. Using pretty much the same formalism (decision trees) applied by emergency room doctors to "triage" desperately ill patients in the ICU, except that the most violent crimes are much more rare than most deadly ailments.
Northpointe is a subsidiary of a Canadian firm, Volaris Group. It has offices in Traverse City, MI and Golden, CO. COMPAS is an acronym for Correctional Offender Management Profiling for Alternative Sanctions, referring to intent to parole convicts who score "low" on the COMPAS recidivism risk score, but the suite includes several other scores.
The buzz-phrase one often hears in the government context is "automated evidence-based decision making". No humans need burden their overstressed brains with hard thought, because the computer can (allegedly) do everything much better than humans.
Governments love COMPAS in part because it promises to relieve them of political pressure to improve conditions in overcrowded prisons, by releasing some prisoners early in a "legally defensible" way. Because, you see, it's all "scientific".
Nevertheless, COMPAS has proven controversial, purely on statistical grounds: it seems that at least two critical academic papers have been published (behind paywalls!), and in addition it seems that some non-public evaluations by various government agencies were also critical. Northpointe has responded to these evaluations with its own papers, which can be found by searching with DuckDuckgo at their website northpointeinc.com.
The academic call and response style of these exchanges is explained by the fact that Northpointe's cofounder Tim Brennan (I'm pretty sure he is no relation to embattled CIA Director and accused war criminal* John Brennan) is a statistician by training, so he is comfortable with academic disputes, and is not afraid to bandy about the terminology of statistics and actuarial science (logistic regression, binary classification, hazard ratios, etc), signal detection theory (ROC curve), machine learning (neural nets, decision trees, support vector machines, etc), and optimization (gradient descent, etc).
In a paper called "Creating Risk-Scores in very imbalanced data sets: Predicting Extremely Violent Crime among criminal offenders following release from prison", Tim Brennan and two of his employees, plus a former colleague from University of Colorado at Boulder, admit that the events the government most wants to predict, whether prospective parolees will commit very violent crimes in the future if they are released early, are very rare, so the Base Rate Fallacy applies in a very strong form. (Likewise, the events NCTC's CVE risk scores try to predict are fabulously rare in the general population.) But Tim Brennan and his coauthors claim that COMPAS can beat the base-rate fallacy.
(See section 5.2 of the paper, which is available as a pdf at northpointeinc.com and is indexed by our favorite search engine, so it should be easy to find. It seems that the paper in question may have been published as a chapter in a book, but I have no print reference.)
I feel sure Brennan et al. are wrong, but if they are right, it would seem Tor might be in trouble, because the arguments are not specific to prediction of any particular type of very rare event.
There is also an issue of network security: clients access their COMPAS suite via a web server which uses Windows 2000/2003 server, SQL 2000/2005, Net 2.0, Asp.net Ajax 2.0. Apparently for ease of information sharing, prisoner's SSNs are exposed in unencrypted data links. Another document indexed by DuckDuckGo suggests that hashing of staff passwords is optional. (Good grief!) Northpointe promises to help local governments turn their pokey into a "smart jail", but I have my doubts about how "smart" this kind of algorithmic decision making really is.
And there is the issue of whether US taxpayers should be funding predictive analysis of very rare events. This stuff is not cheap: Northpointe charged the Josephine County Sheriff's Office (Oregon) $22500 for a COMPAS suite. According to what appears to be the minutes of a public meeting, also available at northpointeinc.com. And that is just one of hundreds of US jurisdictions which have adopted COMPAS.
For layperson-friendly statistical critique of COMPAS from the intrepid data journalists at ProPublica, see
https://www.propublica.org/article/machine-bias-risk-assessments-in-cri…
Machine Bias
There’s software used across the country to predict future criminals. And it’s biased against blacks.
Julia Angwin, Jeff Larson, Surya Mattu and Lauren Kirchner
23 May 2016
http://kwbu.org/post/full-interview-propublicas-julia-angwin-biased-sen…
Full interview: ProPublica's Julia Angwin on biased sentencing algorithms
Bruce Johnson
25 May 2016
> "We looked at risk assessment algorithms used throughout the criminal justice system, and they are often questionnaires about an individual defendant," said Angwin. "They ask about your previous criminal history, your family, and make an assessment of low, medium, or high of whether you would go on to commit a future crime."
>
> ProPublica's analysis found that an algorithm created by the for-profit company Northpointe was only 61 percent accurate in predicting future crime. The analysis also found that the algorithm was twice as likely to give black people a high risk score.
Tip of the iceberg. Female victims of sexual abuse also fare very badly when scored by COMPAS. (You heard that right, being victimized in certain ways sets you up for a lifetime of state-sponsored discrimination by Bayesian risk scoring. Hardly seems fair, does it?) People who live in poor neighborhoods, or have no family ties, are also penalized by COMPAS. It's all right there in Northpointe's own manuals explaining how to interpret their various risk scores.
Statistics matters! Bayesian analysis done badly could very easily become an enabler of American fascism.
I would love to hear comments from everyone who has previously discussed the Base Rate Fallacy in this blog or on tor-talk! (Raccoon?)
*Speaking of predictions, there may be an Interpol warrant in John Brennan's future, but I am too wise--- or too timid-- to assign a probability to this so-far hypothetical possible future event. Stay tuned.